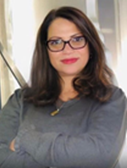
Khadija Rantell
(MHRA)
|
Dr Khadija Rantell is a Senior Statistical Assessor at the MHRA, where she has been working over eight years. Khadija works across divisions (Clinical Trial Unit, Licensing, and Devices).
At the MHRA, she writes assessment reports on methodological aspects of licensing applications, advises companies on methodological aspects of their clinical development programme, and contributes to the assessment of clinical trial applications and clinical investigations of medical devices.
Prior to joining the agency, Khadija worked in academia for over 10 years. She has a PhD in Applied Statistics to Healthcare Research from Sheffield University.
Khadija has a particular interest in early phase clinical trial designs, innovative trial designs, patient reported outcomes, real-word-data, and rare diseases. She is a member of the Patient Reported Outcomes Specialist Interest Group at the MHRA, the EFSPI (European Federation for Statisticians in the Pharmaceutical Industry) Scientific Committee, and the EFPIA/EFSPI Estimand Implementation Working Group (EIWG).
|
Estimands based on composite strategy: examples and regulatory perspectives
The ICH E9 (R1) Addendum introduced the concept of intercurrent events. Intercurrent events may impact the assessment and subsequent interpretation of the outcome of interest. One of the approaches to handling them is the composite strategy, which incorporates intercurrent events into the definition of the endpoint.
Composite endpoints have typically been used as a solution to maintain the feasibility of randomised control trials and to increase statistical efficiency. The composite approach is commonly seen with binary and event-based endpoints, where intercurrent events can often be fairly defined as events or poor outcomes. Examples of proposals where a composite strategy was proposed and regulatory perspectives on these will be presented.
|
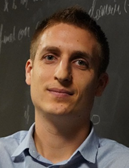
Alex Ocampo
(Novartis Pharma AG)
|
I am currently a Principal Statistician with Novartis in Basel, Switzerland. I obtained my Ph.D. in Biostatistics from Harvard University in 2020 with dissertation titled, “Breaking the MAR Paradigm: Estimation, Bounding, and Sensitivity when Data are Missing Not at Random.” My doctoral dissertation presented novel methods for dealing with missing data when the “Missing at Random” assumption does not hold and encouraged statisticians to look beyond methods leveraging the common assumption. My statistical interests lie primarily in methodologies for dealing with missing data, causal inference, and Bayesian inference. In addition to clinical trial work at Novartis, I am a part of an active collaboration with Oxford University developing novel statistical methodology catered specifically to characterizing disease progression in Multiple Sclerosis. I hail from Ann Arbor, Michigan in the USA where I earned my Bachelor’s degree in Statistics from the University of Michigan.
|
Targeting Composite Estimands using Trimmed Means
A composite estimand is straightforward to construct with a binary outcome in a clinical trial - one treats the intercurrent event (IE) as a failure and absorbs this into the variable definition. The procedure is less clear in the context of continuous outcomes; however, using trimmed means provides one potential path forward. Simply set the "missing values" as the worst observed outcome and then trim away a fraction of the distribution from each treatment arm before calculating differences in treatment efficacy. Because the method penalizes treatment arms with more IE, it provides a composite measure of the treatment’s effect on both the clinical outcome and the IE rate. The drawback of composite estimands lies in their interpretability; therefore, we prove under what assumptions this method can target additional types of estimands. This work elucidates, the often ignored, but important fact, that certain missing data methodologies can be aligned with different estimands depending on the veracity of their assumptions.
|

Samvel Gasparyan (AstraZeneca)
|
Biostatistician with more than 5 years of experience working in the pharmaceutical industry, with a PhD degree in Statistics from Le Man University, France (2016). Primary therapeutic areas of interest are CVRM (cardiovascular, renal and metabolism). Research interests are in statistical aspects of novel types of endpoints, including hierarchical composite endpoints and endpoints based on recurrent events.
|
Design and analysis of studies based on hierarchical composite endpoints: Insights from the DARE-19 trial
Hierarchical composite endpoints (HCEs) are based on clinical outcomes which, unlike traditional composite endpoints incorporate ranking of components according to clinical importance. Design of an HCE requires the clinical considerations specific to the therapeutic area under study and the mechanism of action of the investigational treatment. Statistical aspects for the clinical endpoints include the proper definition of the estimand as suggested by ICH E9(R1) for the precise specification of the treatment effect measured by an HCE.
We describe the estimand of the DARE-19 trial, where an HCE was constructed to capture the treatment effect of dapagliflozin in hospitalized patients with COVID-19, and was analyzed using a win odds. Practical aspects of designing new studies based on an HCE are described. These include sample size, power, and minimal detectable effect calculations for an HCE based on the win odds analysis, as well as handling of missing data and the clinical interpretability of the win odds in relation to the estimand.
|
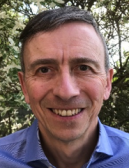
Oliver Keene (KeeneONStatistics Ltd.)
|
Oliver is currently a statistical consultant. He previously worked at GSK for over 25 years in all stages of new medicine development, from the pre-clinical area through to support for reimbursement. He has particular experience of medicines for infectious diseases (influenza, herpes, hepatitis) and respiratory diseases.
|
Discussant
|