Speaker
|
Biography
|
Abstract
|

Jonathan Bartlett,
University of Bath
|
Jonathan Bartlett is a Reader in Statistics at the University of Bath, UK. He worked previously at AstraZeneca’s Statistical Innovation Group, and the London School of Hygiene & Tropical Medicine. His research interests include statistical methods for missing data & estimands, covariate adjustment, survival analysis, and measurement error. He maintains a statistics blog at thestatsgeek.com
|
Non-proportional hazards – an introduction to their possible causes and interpretation.
In this introductory talk I will being by reviewing Cox’s proportional hazards model and the meaning of ‘proportional hazards’. I will illustrate some of the different ways in which non-proportional hazards may arise, and in so doing, demonstrate that interpretation of time-changing hazard ratios is complicated by the fact that the survivors to a particular follow-up time in the two treatment groups will generally systematically differ in respect of baseline prognostic variables.
|
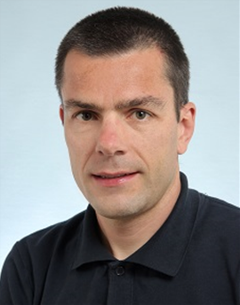
Kaspar Rufibach,
Roche
|
Kaspar Rufibach is a member of Roche's Methods, Collaboration, and Outreach group and located in Basel. He does methodological research, provides consulting to Roche statisticians and broader project teams, gives biostatistics trainings for statisticians and non-statisticians in- and externally, mentors students, and interacts with external partners in industry, regulators, and the academic community in various working groups and collaborations. He has co-founded and co-leads the European special interest group “Estimands in oncology” (sponsored by PSI and EFSPI) that currently has more than 35 members from 22 companies and several Health Authorities and works on various topics around estimands in oncology. Kaspar’s research interests are methods to optimize study designs, advanced survival analysis, probability of success, estimands and causal inference, estimation of treatment effects in subgroups, and general nonparametric statistics. Before joining Roche, Kaspar received training and worked as a statistician at the Universities of Bern, Stanford, and Zurich.
|
Planning a Phase 3 trial with time-to-event endpoint, a cure proportion, and a futility interim analysis using response.
With median overall survival (OS) of about six months and no approved drug for more than forty years, the unmet medical need in acute myeloid leukemia is dramatic. Idasanutlin is a MDM2 antagonist that can effectively displace p53 from MDM2 to restore p53 function, leading to cell cycle arrest and apoptosis of cancer cells. Planning the Phase 3 trial MIRROS comparing Idasanutlin + standard of care against the standard of care presented with the following challenges: (1) To survive AML a patients needs to become eligible for a bone marrow transplant, trough achieving a complete response (CR) after induction therapy. Planning the trial using overall survival as primary endpoint thus needs to account for a cure proportion in both, the treatment and control arm. (2) MIRROS was planned based on Phase 1 data only. To mitigate the risk of directly moving to Phase 3, a futility interim was built in the design, using gates on the odds ratio for. The interim analysis was built-in the design using a mechanistic simulation model, making assumptions on response proportions, proportion of transplant survivors, and OS in these various groups. The talk describes the design in detail, discusses sample size planning, operating characteristics of the futility interim analysis, and will touch upon how we plan to report the results. We conclude with sharing feedback from US and European Health Authorities on the design.
|
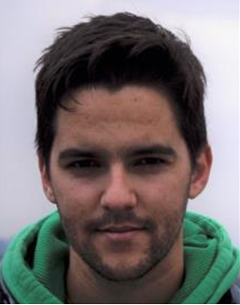
Jose Jimenez,
Novartis
|
With a PhD in Statistics from Politecnico di Torino, Jose participated as an Early Stage Researcher in the Marie Curie network “IDEAS”, where he primarily worked on Bayesian dose finding methods and non-proportional hazards. He is currently employed by Novartis in Basel.
|
Evaluating the impact of delayed effects in confirmatory trials.
The presence of delayed effects causes a change in the hazard ratio while the trial is ongoing since at the beginning we do not observe any difference between treatment arms, and after some unknown time point, the differences between treatment arms will start to appear. The weighted log-rank test allows a weighting for early, middle, and late differences through the Fleming and Harrington class of weights and is proven to be more efficient when the proportional hazards assumption does not hold. We explore the impact of delayed effects in group sequential and adaptive group sequential designs and make an empirical evaluation in terms of power and type-I error rate of the of the weighted log-rank test. We also give some practical recommendations regarding which methodology should be used in the presence of delayed effects depending on certain characteristics of the trial.
|
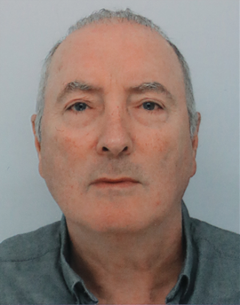
John O’Quigley,
UCL
|
John started his career at the University of Leeds before moving to France in the mid eighties. In the late eighties, he worked as Associate Professor of Biostatistics at the University of Washington, Dept of Biostatistics and the Fred Hutchinson Cancer Research Center in Seattle. Throughout the nineties until 2004, he resided as Full Professor of mathematics at the University of California San Diego. From 2006 until 2010 he was Full Professor of Biostatistics at the University of Virginia, since which time he was full professor at the University of Paris-Sorbonne until the end of 2018. In 2019 he became full professor in the Dept of Statistical Science, University College London.
|
Constructing survival models and testing effects in non-proportional hazards situations.
We describe a unified framework within which we can build survival models. Our focus is on how to best code, or characterise, the effects of the variables, either alone or in combination with others. We consider simple graphical techniques that not only provide an immediate indication as to the goodness of fit but, in cases of departures from model assumptions, point to the form of a more involved non-proportional hazards model.
One advantage, similar to a linear regression scatterplot, is that no estimation is required. These graphical techniques help support our intuition. This intuition is backed up by formal theorems that underlie the process of building richer models from simpler ones. Goodness-of-fit techniques are used alongside measures of predictive strength and, again, formal theorems show that these measures can be used to help identify models closest to the unknown non-proportional hazards mechanism that we can suppose generates the observations.
We consider many examples and show how these tools can be of help in guiding the practical problem of efficient model construction for survival data as well as for carrying out formal statistical tests, with good power properties, in situations of non-proportional hazards.
|

Satrajit Roychoudhury,Pfizer
|
Dr. Satrajit Roychoudhury is a Senior Director and a member of Statistical Research and Innovation group in Pfizer Inc. Prior to joining, he was a member of Statistical Methodology and consulting group in Novartis. He started his career as a research statistician in Schering Plough Research Institute (now Merck Co.). He has 12+ years of extensive experience in working with different phases of clinical trial. His primary expertise includes implementation of innovative statistical methodology in clinical trial. He has co-authored several publications/book chapters in this area and provided statistical training in major conferences. His area of research includes survival analysis, use of model based approaches and Bayesian methods in clinical trials. Satrajit was a recipient of a Young Statistical Scientist Award from the International Indian Statistical Association in 2019.
|
A Robust Design Approach for Clinical Trials with Potential Non-proportional Hazards: A Straw Man Proposal.
Targeting the immune system to cure cancer has emerged as a promising treatment option for patients in recent years. Instead of targeting a tumor directly or destroying it with radiation, Immunotherapy boosts the body's natural defenses to fight cancer. However, this novel treatment poses new challenges in the study design and statistical analysis of clinical trials. A major challenge is the delayed onset of treatment effects due to the mechanism of immunotherapy which violates the proportional hazard (PH) assumption. The conventional log-rank test may suffer a significant power loss in such scenarios. It is often referred as the non-proportional hazard (NPH) problem. In contrast to the PH assumption, NPH constitutes a broad class of alternative hypotheses. While there may be speculation about the nature of treatment effect at the time of study design, we have found it can often be wrong. Therefore, designing a trial that will be well-powered and adequately describe the treatment effect over time is often challenging. A suitable design for time to event data with potential NPH needs to be flexible enough to incorporate the uncertainty of NPH type and provide a robust inference. Often a trial involves interim analysis for early stopping due to futility or overwhelming efficacy. Group sequential methods are popularly used in this context. Although group sequential strategies are well understood using the log-rank test in the PH setting, little attention has been given to their performance when the effect of treatment varies over time.
This presentation will focus on an alternative design approach for immune-oncology trials. The proposed design approach is based on a combination of multiple Fleming-Harrington WLR tests and is referred as the MaxCombo test. It chooses the best test adaptively depending on the underlying data. The main objective the new design is to provide robust power for primary analysis under different NPH scenarios. The talk will provide the general design framework, sample size calculation, and evaluation of operating characteristics. In addition, a comparison of MaxCombo with other available approaches will be provided. Finally, It will reflect on further extensions of the MaxCombo test in group sequential design. A real-life example will be used for illustration.
|
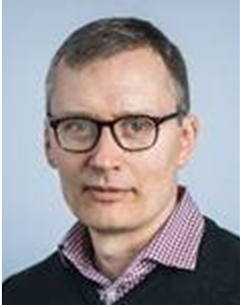
Carl-Fredrik Burman,
AstraZeneca
|
Carl-Fredrik “Caffe” Burman is Senior Statistical Science Director at AstraZeneca, where he has been working for a quarter of a century. He is part of the methodology group, Statistical Innovation, and works mainly on internal design consultations. Caffe is currently co-leading a project on Innovative Trial Designs for Oncology trials. He is an adjunct professor at Chalmers Univ.
|
Inference in survival trials: Weighted log-rank tests and some alternatives.
We can do a lot with standard t-tests, ANCOVA and Wilcoxon. Does it have to be way more complicated if we have time-to-event data? And how can we handle trials where the relative efficacy changes over time? We will revisit some basic inference theory to generate ideas for how to test for benefit when mean survival functions may be crossing. It is demonstrated that many weighted logrank tests do not control the relevant type 1 error.
We have therefore developed the Modestly Weighted Logrank Test with
1) superior power compared with the standard unweighted logrank test when PD-1/PD-L1 inhibitors are compared to chemotherapy, and
2) strong error control not only when survival functions are identical but also when survival may be higher for control.
|
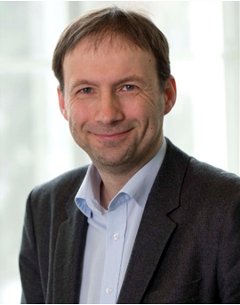
Martin Posch,
Medical University of Vienna
|
Martin Posch is professor of medical statistics at the Medical University of Vienna and head of the Center for Medical Statistics, Informatics and Intelligent Systems. From 2011-2012 he worked as statistical expert at the European Medicines Agency (London, UK) in the Human Medicines Development and Evaluation sector, where he contributed to guideline development and the assessment of study designs. He has a PhD in Mathematics from the University of Vienna and was scientific assistant and associate professor at the Medical University of Vienna. His research interests are group sequential trials, adaptive designs and multiple testing, focusing on applications in clinical trials and Bioinformatics. Martin Posch serves as Associate Editor of Biometrics and Biometrical Journal and is currently member of the executive board of the Austro-Swiss Region of the International Biometric Society and Observer of the EMA Biostatistics Working Party.
|
Confirmative assessment of differences in the survival function based on multiple characteristics.
If the proportional hazards assumption does not hold the standard hazard ratio estimate is not a reliable measure of the treatment effect: it depends not only on the actual survival distributions but also on the censoring pattern, the study duration, and variations in the recruitment rates. Furthermore, in case of crossing hazard or survival functions estimated hazard ratios are hardly interpretable.
However, under non-proportional hazards, differences in the survival functions can be described by several parameters such as the difference between survival probabilities at predefined time points (as 1-year and 2-year survival) the difference between quantiles of the survival functions (as the difference in medians), or average hazard ratios computed up to a predefined time-point. Which of these parameters is best suited to quantify the difference in the survival functions can depend on their specific shape. Therefore, it can be desirable to specify more than one of these parameters as primary endpoint. However, if more than one primary endpoint is considered, a multiplicity problem arises and an inference approach controlling the family wise type I error rate as well as simultaneous coverage of confidence intervals are required for confirmatory conclusions.
Based on the counting process representation of survival function estimators, we show that the considered estimators are asymptotically multivariate normal and derive an estimator of their asymptotic covariance matrix and resulting asymptotic simultaneous confidence intervals. Furthermore, as alternative method, we derive simultaneous confidence intervals based on the perturbation approach for survival function estimates, which corresponds to a parametric bootstrap.
The finite sample properties of the proposed methods are investigated in a simulation study. We find that coverage probabilities are close to the nominal value, even for moderate sample sizes.
|