Speaker
|
Biography
|
Abstract
|
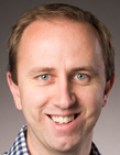
Jonathan Bartlett
(London School of Hygiene & Tropical Medicine)
|
Jonathan Bartlett is a Professor in Medical Statistics at the London School of Hygiene & Tropical Medicine. His research interests are focused around missing data and causal inference methods, and more recently, how these can be applied to target different estimands in clinical trials. He has held previous positions at AstraZeneca and the University of Bath, and maintains a blog https://thestatsgeek.com/
|
An introduction to covariate adjustment in trials.
In this introductory talk I will begin by describing the motivations for covariate adjustment in randomised trials. I will then describe the usual way a covariate adjusted analysis is performed, namely via fitting a suitable outcome regression model, highlighting the type of estimand such an approach targets and the statistical assumptions it relies on. Next, I will describe the standardisation or G-computation approach to covariate adjustment, as described in the FDA’s covariate adjustment guidance. I will contrast it with the usual approach in terms of efficiency, robustness and in terms of the estimand it targets.
|
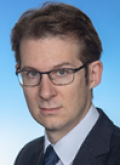
Florian Voss (Boehringer Ingelheim)
|
Florian Voss is Expert Therapeutic Area (TA) Statistician at Boehringer Ingelheim. He works in all phases of clinical development and provides statistical consultation and advice for the statisticians working for TA Inflammation at BI. His is interested in applications of statistical methods in clinical development, e.g. paediatric extrapolation, covariate adjustment, interim analyses and inclusion of historical data.
|
Summary of regulatory guidances (FDA, EMA) on covariate adjustment and comments from EFSPI/PSI Regulatory SIG.
In many clinical trials there are known baseline factors that are prognostic or predictive for outcomes in the trial. It is important to suitably account for such covariates in the planning and statistical analysis of a clinical trial to improve sensitivity for treatment effects, and to minimise the impact of any chance baseline imbalances between arms.
Regulatory agencies generally support inclusion of covariates in the primary analysis model. The ICH E9 guidance recommends to identify covariates that have an important influence on the primary endpoint and to account for them in the analysis. More detailed guidance is provided in the EMA “Guideline on adjustment for baseline covariates in clinical trials” from 2015 and the more recent FDA Guidance for the Industry “Adjusting for Covariates in Randomized Clinical Trials for Drugs and Biological Products” from 2021.
This presentation will summarize the available regulatory guidance and the comments provided by the EFSPI/PSI regulatory SIG. We will review the difference between conditional and unconditional treatment effects for linear and some non-linear models and discuss them in the context of ICH E9 (R1) “Estimands and Sensitivity Analysis in Clinical Trials”.
|
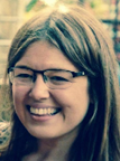
Rhian Daniel
(Cardiff University)
|
Rhian Daniel is Professor of Statistics at the Division of Population Medicine, Cardiff University. Her research interests include several areas of causal inference methods and their application.
|
Marginal versus conditional effects and collapsibility.
This presentation will start with the difference between marginal and conditional estimands, and settings in which one or the other may be more pertinent to the clinical question of interest. The distinction between marginal and conditional estimands will be contrasted with that between unadjusted and adjusted analyses. The phenomenon of non-collapsibility will then be discussed in detail, including an intuitive explanation of what it is and situations in which we should be mindful of it.
|
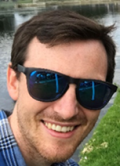
Tim Morris
(MRC Clinical Trials Unit at UCL)
|
Tim Morris is a principal research fellow based at the MRC Clinical Trials Unit at UCL. He works on the development, evaluation and understanding of statistical methods. His interests include simulation studies, handling missing data, sensitivity analysis, covariate adjustment, estimands, and IPD meta-analysis.
|
Planning a covariate adjustment method for your SAP.
It has long been advised to adjust for covariates in the analysis of phase III clinical trials. The key justifications are: 1) adjustment increases power; 2) when randomisation was stratified, ignoring covariates in the analysis leads to incorrect estimates of uncertainty (such as confidence intervals that are too wide).
In practice we need to define how we will adjust for covariates in a statistical analysis plan. The usual approach is direct adjustment, where an outcome regression model includes the covariates and the treatment effect is estimated as a parameter of this model. Two alternative approaches that have received less attention from the trials community are standardisation and inverse-probability-of-treatment weighting (IPTW).
This presentation will compare the three broad approaches in terms of planning which to write into a SAP. Beginning with the summary measure attribute of the estimand, I will discuss points to consider such as efficiency, variance estimation, convergence issues, and handling of missing data. No single approach is best for all situations but standardisation and IPTW deserve far more consideration than they currently receive.
|

Seth Seegobin (AstraZeneca)
|
Seth Seegobin is the Head of Biostatistics, Vaccine and Immune Therapies at AstraZeneca. His statistical interests include programming efficient simulations, interim analyses and the use of sampling error during trial design.
|
Immunogenicity and prognostic effects.
Immunobridging trials aim to infer vaccine effectiveness (in the absence of efficacy data) by comparing the immune response of a vaccine candidate with an approved vaccine.
Within an RCT, typically we rely upon randomisation to safeguard against treatment arm imbalance for important response prognostic variables, however the prioritisation of demographic groups within national vaccine programmes can create several challenges for immunobridging trials that follow.
This presentation will summarize the challenges and possible solutions to accurately describe and compare immunogenicity between booster and primary series treatment arms, imbalanced with respect to prognostic baseline characteristics, using covariate adjustment.
|