PSI Sub Group SIG Webinar: Modern approaches to subgroup identification
Date: Wednesday 17th November 2021
Time: 15:00-17:00 GMT
Speakers: David Svensson, Björn Bornkamp, Ilya Lipkovich, Kostas Sechidis and Paolo Eusebi
Who is this event intended for? A broad audience of statisticians and data scientists.
What is the benefit of attending? Attendees will get an overview from experts to a rather technical area. They'll also gain an understanding of what the latest trends are in this research via a mixture of theory & practice.
Overview
In this webinar we look at some recent advances in statistical methods for identifying treatment effect heterogeneity in clinical trials. This ranges from identifying baseline biomarkers likely to influence the treatment effect (ranking) to provide novel biomarker 'signatures' (subgroups) with associated estimated enhanced effect (Individual Treatment Effects). Some practical issues ranges from overfitting risks, biases, and confounding of prognostic and predictive effects. Modern methods aim to overcome such potential difficulties while remaining flexible, and offer a structured approach to the problem (aiming to avoid the notorious 'data dredging'). The novel techniques are often tree based and/or penalized regression, i.e., with a machine learning flavour. Sometimes the aim of the analysis is to predict the individual optimal treatment allocation given baseline biomarker data (Individual Treatment Rules). Efficient Visualization of relationships in the data is also of importance in the practical applications. The talks will highlight and discuss such aspects and will also reflect typical aspects discussed within the EFSPI/PSI Subgroup Special Interest Group. (While this event is not intended as a formal course, it will still serve as an introduction and overview to the area, as well as covering some more technically challenging material for the more experienced participant).
Registration
This webinar is free of charge to both Members and Non-Members of PSI.
To register your place, please click here.
Speaker Details
Speaker
|
Biography
|
Abstract
|
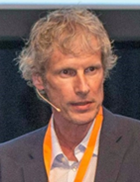
David Svensson, SIG lead
(AstraZeneca)
|
Senior Statistical Science Director at the Statistical Innovation group, Data Science and AI, Gothenburg. Has a Ph.D. in Mathematical Statistics from Chalmers University of Technology, Sweden and was Post Doc at the University of Zurich & ETH in 2000-2003, working & teaching in the Bioinformatics field. Joined AstraZeneca R&D in 2003, with involvement ranging from Discovery science to Late Phase drug development, with a particular interest in exploratory statistical modelling and machine learning. Current Lead of the EFSPI Subgroup Special Interest Group.
|
Introduction
Introducing the SIG and discussing the taxonomy of the topics falling under the SIG umbrella. A short overview of the various flavors of subgroup analyses in pharmaceutical drug development e.g., confirmatory testing, regulatory Consistency assessment, subgroup selection and detection using Causal Inference Machine Learning (ITE/ITR approaches).
|
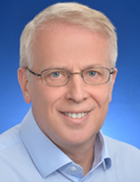
Ilya Lipkovich
(Eli Lilly)
|
Ilya Lipkovich is a Sr. Research Advisor at Eli Lilly and Company. Ilya received his PhD in Statistics from Virginia Tech in 2002 and has more than 15 years of statistical consulting experience in pharmaceutical industry. He is an ASA Fellow and published on subgroup identification in clinical data, analysis with missing data, and causal inference. He is a frequent presenter at conferences, a co-developer of subgroup identification methods, and a co-author of the books “Analyzing Longitudinal Clinical Trial Data. A Practical Guide” and “Estimands, Estimators and Sensitivity Analysis in Clinical Trials.”
|
Overview of recent innovations in subgroup identification for personalized medicine and related methods
Ilya Lipkovich (Eli Lilly) and Alex Dmitrienko (Mediana)
In this talk we review recent advances in statistical methods for personalized medicine including subgroup identification (SI) and estimation of individualized treatment regimens (ITR) from randomized clinical trials and observational data. We identify several types of approaches using features introduced in Lipkovich, Dmitrienko and D’Agostino (2017) that distinguish principled methods from various simplistic approaches to data-driven subgroup identification and discuss common measures for evaluating performance of SI and ITR.
|
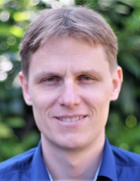
Björn Bornkamp
(Novartis)
|
Björn Bornkamp works since 10 years in the Statistical Methodology Group at Novartis in Basel, where he provides consulting to statisticians and clinical teams on topics related to dose-finding studies, subgroup analyses, Bayesian statistics as well as estimands and causal inference. Björn holds a PhD in Statistics from TU Dortmund University in Germany. He received the RSS/PSI award for developing statistical dose-finding methodology, in particular the DoseFinding R package
|
Benchmarking algorithms for discovering subgroups with differential treatment effect
The identification and estimation of heterogeneous treatment effects in biomedical clinical trials is challenging because trials are typically planned to demonstrate an effect in the overall trial population. Nevertheless, the identification of how the treatment effect may vary across subgroups is of major importance for drug development. In this work, we review and benchmark recent methods for identifying and estimating the heterogeneous treatments effects using various metrics and scenarios relevant for drug development. Our focus is not only on a comparison of the methods in general, but on how well these methods perform in realistic simulation scenarios.
|
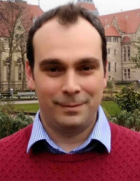
Kostas Sechidis
(Novartis)
|
Kostas is an Associate Director of Data Science in Novartis’ Advanced Exploratory Analytics group. His main areas of interest are machine learning based biomarker discovery, subgroup identification, and development of digital endpoints. Kostas did his PhD in statistical machine learning on the area of hypothesis testing and feature selection in semi-supervised scenarios in the University of Manchester’s Department of Computer Science. Afterwards, he spent many years as post-doctoral researcher on developing novel methodologies for analysing: self-reported epidemiological data with Manchester’s Health e-Research Center, clinical trials data for personalised medicine with AstraZeneca and digital healthcare data for digital biomarker development with Roche. More information about his work can be found at: https://sechidis.netlify.app/
|
Using knockoffs for controlled predictive biomarker identification
One of the key challenges of personalized medicine is to identify which patients will respond positively to a given treatment. The first step towards this is to identify the baseline variables (e.g. biomarkers) that influence the treatment effect, which are known as predictive biomarkers. When we discover predictive biomarkers it is crucial to have control over the false-positives to avoid waste of resources, as well as provide guarantees over the replicability of our findings. With our work we introduce a set of methods for controlled predictive biomarker discovery, and we use them to explore heterogeneity in psoriatic arthritis trials.
|
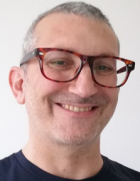
Paolo Eusebi
(UCB Pharma)
|
Eusebi is a Statistician and Statistical Programmer. After obtaining a PhD in Mathematics and Statistics in 2007, he has worked as a statistician both in academia and in public health sector. Since 2019 he joined UCB and the pharmaceutical industry. His main research interests are evidence synthesis, patient reported outcomes, statistical learning techniques for subgroup identification, and data visualization. He is Senior Editorial Board Member of BMC Medical Research Methodology and an active member of PSI and Data Visualization Society.
|
Visualize and interact with subgroups
Graphical approaches are routinely employed in subgroup analysis, typically for describing treatment effect sizes of subgroups. Such graphical displays could be either static or interactive, escriptive or exploratory. Such visualization techniques could be handsome in summarizing a pre-specified analysis, or they could serve as an exploratory tool. One example of an interactive exploratory tool is the subscreen R package. A mini review of such graphical approaches to subgroup problems is presented. Furthermore, we will introduce a novel tool for the exploration of predictive factors of safety and efficacy in a pool of clinical trials.
|