PSI Scientific Committee Webinar - Longitudinal modelling: Time to take the next step?
Date: Monday 18th November 2019
Time: 14:00 - 16:00 UK Time
Speakers:
- José Pinheiro
- Björn Bornkamp
- Tobias Mielke
- France Mentré
- Rob Hemmings
Post-event access: To access the recording of this webinar, please visit the Video On Demand library.
Registration: This webinar is free for PSI members, but has a charge of £20+VAT for non-members. To register, please click here.
Please email PSI@mci-group.com if you have any questions.
___________________
Longitudinal data, i.e. data that arises from repeated observations of the variable over a period of time, has long been put forward as one way to improve to the efficiency of drug development. However, even though there is a rich statistical methodology for longitudinal data, there is no full, wholehearted uptake of these methods in pharmaceutical statistics. The purpose of this webinar is to explore the use of longitudinal modelling across drug development, highlighting its opportunities (such as usage as primary analyses, or for improved decision making at interim analyses) and caveats. One important aspect to be discussed is the evaluation of the efficiency of (parametric) longitudinal modelling versus standard cross-sectional approaches, and factors based upon which one approach might be preferable over the other.
Speakers

José Pinheiro
(Janssen Research & Development)
|
Abstract: Increasing the efficiency of drug development is imperative to make novel treatments available to patients sooner and at lower costs, ensuring the long-term sustainability of the biopharmaceutical industry. Among the various proposals that have been put forward to improve drug development efficiency, leveraging longitudinal data via (parametric) modeling stands out for its low additional cost and ease of implementation: oftentimes only involving different analysis methods for data already collected in clinical studies. While numerous statistical approaches have been developed over the past several decades for modelling longitudinal data (e.g. nonlinear and generalized linear mixed effects) the uptake of these methods in pharmaceutical statistics as part of mainstream (pre-specified) primary analyses in clinical trials remains quite limited, with main exception being MMRM.
Partially this may be due to concerns about making assumptions on the shape of longitudinal profiles, leading to the focus on cross-sectional (or landmark) analyses, which do not require such assumptions, but often only utilize a fraction of the information available per patient. By utilizing the full information collected over time, longitudinal modelling, especially of the parametric type, has the potential to lead to substantially more efficient drug development, even when the primary endpoint is cross-sectional in nature (e.g., change from baseline at Week 26). This potential advantage needs to be balanced against standard concerns about model mis-specification and regulatory acceptance.
Bio: José Pinheiro has a Ph.D. in Statistics from the University of Wisconsin – Madison, having worked at Bell Labs and Novartis Pharmaceuticals, before his current position as Global Head of Statistical Modeling & Methodology in the Statistics and Decision Sciences department at Janssen Research & Development. He has been involved in methodological development in various areas of statistics and drug development, including dose-finding, adaptive designs, and mixed-effects models. He is a Fellow of the American Statistical Association, a past-editor of Statistics in Biopharmaceutical Research, and past-president of ENAR.
|
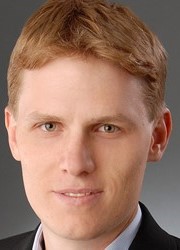
Björn Bornkamp
(Novartis)
|
Title: When is a longitudinal test better than a cross-sectional one for detecting a treatment effect?
(Joint work with Ines Paule)
Abstract: In this work, we explore the potential benefits of utilizing the full longitudinal profile for testing for a treatment effect and compare this to a cross-sectional test at the last time point.
While there are a number of papers showing huge gains for longitudinal testing, current practice in clinical development is to focus on a comparison at the last visit for the purpose of trial design and the primary statistical analysis. In this presentation we try to characterize the factors and endpoint properties that determine if and by how much a longitudinal test will be more powerful than a cross-sectional test.
We consider the setting of a continuous endpoint measured repeatedly over time and utilize a test that uses a weighted average of the treatment differences at the specific time points, which is straightforward to implement with standard mixed effects software. We consider how to weight time-points optimally and which factors play a role in determining the weights. We then assess the potential gain of the longitudinal approach in a set of real case examples.
Finally, a simulation study is performed that compares this simple longitudinal approach to more strongly parameterized traditional longitudinal mixed effects model.
Bio: Björn works in the Novartis Statistcal Methodology and Consulting group. He consults for example on dose-finding studies, causal inference and estimands, subgroup analysis, Bayesian statistics and statistical modelling. In 2013 he received the RSS/PSI award for developing innovative statistical dose-finding methodology, in particular the development of the software package DoseFinding in R.
|
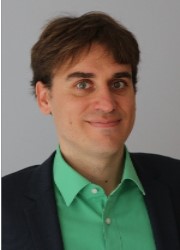
Tobias Mielke
(Janssen Research & Development)
|
Title: Decision-making using longitudinal modelling in presence of model uncertainty
Abstract: As statisticians, we design studies to answer some research questions and we would like to get answers to these research questions as quickly as possible, using the least amount of resources while still providing enough information to allow for some “significant” conclusions with high probability. It is common that our study designs require multiple assessments of the same variable within the same subjects over some period of time. However, while these correlated longitudinal measurements are collected, our analysis approaches might not take full use of the information contained in the data, leading to inefficiency in some of our analyses and potentially to wrong decisions. Many statistical techniques are available to leverage the information contained in longitudinal data, like summary measures (e.g. AUCs) or the MMRM approach. Knowing the true underlying longitudinal profile and distribution, parametric longitudinal modelling provides an efficient analysis technique. Unfortunately, the true underlying longitudinal profile is known only in rare situations (e.g. mechanistic models in PK/PD). As a result, high uncertainty in longitudinal profiles comes with high concerns in applying parametric longitudinal modelling for decision-making: Using the wrong model for the analysis, the results will be biased such that error probabilities will be inflated. While model uncertainty is a valid concern, mitigation strategies should be evaluated in the design phase to support the selection of an efficient and robust analysis technique.
Concerns on model uncertainty have been widely discussed for dose-finding studies in the recent scientific literature. The methodology can be generalized to longitudinal modelling, covering model selection approaches, model-based contrast tests and/or (Bayesian) model averaging approaches. The effects of model uncertainty on decision-making will be discussed in this presentation. Different parametric and semi-parametric longitudinal modelling approaches will be evaluated for this purpose. The presented approaches will be compared in their ability of mitigating concerns on the true underlying longitudinal model, while increasing efficiency in decision-making.
Bio: Tobias works as Scientific Director in Janssen’s internal statistical consulting group. His primary consultancy responsibilities are on adaptive study designs, the handling of multiplicity and statistical modelling in general. Tobias joined Janssen in 2018 from ICON Clinical Research, where he implemented adaptive dose ranging designs, including MCP-Mod, into ADDPLAN DF. In his consultancy roles at ICON and Janssen, he supported many innovative study designs projects, including: inferentially seamless Phase 2/3 designs, adaptive Phase 2 Dose-Finding designs with MCPMod, Phase 1/2 PoC Dose-Finding designs using Bayesian Go/No-Go criteria and designs with adaptive endpoint selection. Tobias holds a PhD degree from Otto-von-Guericke-Universität Magdeburg in Germany. His doctoral dissertation was on the topic of optimum experimental design for nonlinear mixed effects models.
|
Discussants
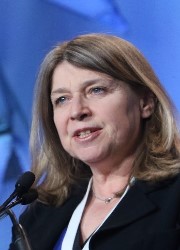
France Mentré
(School of Medicine of University of Paris)
|
Bio: France Mentré is Professor of Biostatistics in the School of Medicine of University of Paris. She heads an INSERM research team on Biostatistical Modelling and Pharmacometrics in treatment of Infectious Diseases. She has worked on development and application of methods for nonlinear mixed-effects models and pharmacometrics for more than 30 years. She applies these models to understand the variability in the response to anti-infective agents. She is leading the development of the software PFIM for optimal design in pharmacometrics. She has published more than 250 articles in biostatistics, pharmacometrics, clinical pharmacology or medical research.
She received in 2013 the USCF/ISoP Lewis B. Sheiner Lecturer Award and in 2018 the ASCPT Sheiner-Beal Pharmacometrics award. She is the co-chair and one of the founder of the Special Interest Group on Statistics and Pharmacometrics of ASA and ISOP. She is editor in chief since October 2018 of CPT: Pharmacometrics and System Pharmacology.
|

Rob Hemmings
(Consilium)
|
Bio: Rob Hemmings is a partner at Consilium. Consilium is his consultancy partnership with Tomas Salmonson, a long-standing member of the EMA’s CHMP and formerly the chair of that committee. Tomas and Rob support companies in the development, authorisation and life-cycle management of medicines.
Previously Rob worked at AstraZeneca and for 19 years at the Medicines and Healthcare products Regulatory Agency, heading the group of medical statisticians and pharmacokineticists. He is a statistician by background and whilst working at MHRA he was co-opted as a member of EMA’s CHMP for expertise in medical statistics and epidemiology. At CHMP he was Rapporteur for multiple products and was widely engaged across both scientific and policy aspects of the committee’s work. He was fortunate to chair the CHMP’s Scientific Advice Working Party for 8 years and also chaired their expert groups on Biostatistics, Modelling and Simulation and Extrapolation. He wrote or co-wrote multiple regulatory guidance documents, including those related to estimands, subgroups, use of conditional marketing authorisation, development of fixed-dose combinations, extrapolation and adaptive designs. He has a particular interest in when and how to use data generated in clinical practice to support drug development.
|