PSI Webinar: Avoiding Pitfalls in Supervised/Unsupervised Learning
Time: 14:00 - 15:30 UK Time
Presenters: Ilya Lipkovich (IQVIA), Alexander Schacht (Lilly) and Andy Nicholls (GSK)
As the availability of big data increases and statisticians assist with predicting outcomes or understanding patterns in an ever-wider variety of scenarios then supervised and unsupervised learning methods become increasing called upon. Such machine learning algorithms offer the opportunity to understand potential predictors or clusters amongst large datasets, but are also subject to the risks of overfitting or over-interpretation. This Webinar seeks to introduce ideas and share experiences in this field.
The talks will introduce several supervised and unsupervised learning methods and cover data-driven subgroup identification in clinical trials, and case studies of implementation clustering algorithms.
Abstracts
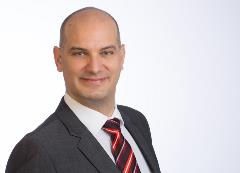
Alexander Schacht, Lilly |
Not all patients are created equal, but are there subgroups that are more homogenous?
Abstract: Can I divide my overall patient population into meaningful segments? Do patients follow different patterns over time? We should ask these questions more often and techniques of unsupervised learning, where the classification of a patient into a group is unknown, answers these questions. We differentiate these approaches from supervised learning techniques in which classification of the patients is known. Typical questions for supervised learnings algorithms include: Can I predict patients outcomes given his/her baseline characteristics?
Cluster analysis represents a class of approaches in unsupervised learning. It helps to answer the above questions. Cluster analysis stands on the determination of metrics, which measure the distances between patients in terms of their many different characteristics. In this presentation, I will present and discuss different approaches available in SAS.
The determination of the number of clusters represents a classical problem of bias-variance trade-off. The presentation will discuss various heuristics but also practical considerations to determine a reasonable choice of clusters.
The practical implementation of cluster analyses comes with various challenges. I will discuss standardization of variables, weighting of variables, correlated data, outliers, finding spurious small clusters, and identification of relevant clusters.
Finally, the communication of cluster analyses has its unique challenges and I will mention various approaches based on real case studies.
Bio: Alexander Schacht (PhD), Principal Research Scientist, Global Statistical Sciences leads a group of 5 European based statisticians driving the statistical activities around launch preparation including HTA submission to support access and commercialization in different auto-immune diseases. After 2 years at Boehringer Ingelheim, Alexander joined Lilly in 2004 and held various positions within statistics with a focus on neurosciences working on phase I, III, and IV in areas like Alzheimer, Schizophrenia, ADHD, Depression, and Pain. Alexander received his PhD in Biometrics in 2002 from the University of Göttingen on work related to non-parametric analysis of covariance. For the publication based on this, he was awarded the 1st. Gustav-Adolf-Lienert Price in 2009 by the German region of the International Biometrical Society. He has published both methodological papers (e.g. on network-meta-analysis, non-inferiority approaches for time-to-event data) and medical papers including more than 60 papers in peer-reviewed biomedical journals. He is a regular speaker at both medical and statistical international conferences. As the chair of the special interest group on benefit-risk of the European Federation of Statisticians in the Pharmaceutical Industry, Alexander is leading and promoting research on quantitative assessments of benefit-risk. He is interested in all aspects of launching new treatments.
|
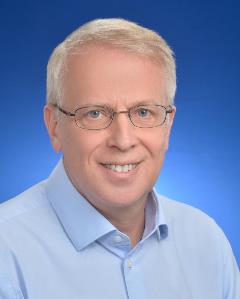
Ilya Lipkovich, IQVIA |
Overview of methods for subgroup and biomarker identification from clinical data
Abstract: In this talk I will provide a high-level description of a broad class of statistical methods for subgroup/biomarker identification in early and late-phase clinical trials. First, I contrast “data-driven” subgroup analysis with a traditional “guideline-driven” approach and describe key elements of principled data-driven subgroup analysis. Then I review 4 classes of methods for subgroup identification that had emerged recently as a result of cross-pollination across machine learning, causal inference and multiple testing (global outcome modeling, global treatment effect modeling, modeling individual treatment regimes, and local treatment effect modeling). I also briefly review available software and key features of subgroup identification methods.
Bio: Ilya Lipkovich is a Sr. Research Advisor at Eli Lilly working in Real World evidence. He received his Ph.D. in Applied Statistics from Virginia Polytechnic Institute and State University in 2002. He has more than 15 years of statistical consulting experience in pharmaceutical industry. Dr. Lipkovich research interests include subgroup identification in clinical data, analysis with missing data, and causal inference from observational data. He is a chair a Subgroup Analysis Working Group sponsored by the Society of Clinical Trials. He has published widely including co-authoring a book “Analyzing Longitudinal Clinical Trial Data. A Practical Guide.”
|
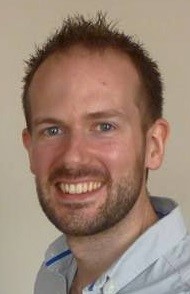
Andy Nicholls, GSK |
Using the SIDES algorithm to the identify patient phenotypes that have the potential to benefit most from switching to Relvar
Abstract: In 2016 GSK successfully completed the Salford Lung Study, a 12-month, open label, randomised, effectiveness study to evaluate fluticasone furoate (FF, GW685698)/vilanterol (VI, GW642444) Inhalation Powder delivered once daily via a Novel Dry Powder Inhaler (NDPI) compared with the existing COPD maintenance therapy alone in subjects with Chronic Obstructive Pulmonary Disease (COPD).
Upon completion of the study, the Scientific Committee expressed an interest in using a data-driven approach in order to identify patient subgroups for which the treatment effect was strongest. In this presentation we will look at why SIDES was chosen for this analysis, the design parameters, and how it fared.
Bio: Andy is a Statistician with a strong interest in Data Science, having previously worked as a specialist R Consultant and Data Scientist for Mango Solutions. On re-joining GSK in 2017, Andy provided support to the Relvar project, for which he led an exploratory cluster analysis using Salford Lung Study data in order to try to identify patient subgroups that might experience an additional real-world benefit of Relvar. He now works in GSK’s new Statistical Data Sciences division within BioStats and is Business Systems Owner for the BioStats HPC environment for R.
|
Click
here to view the flyer.
PSI Member |
Free |
Non-member |
£20 (plus VAT) |
Registration has now closed.